Balancing AI Innovation with Environmental Sustainability
Published on March 07, 2025
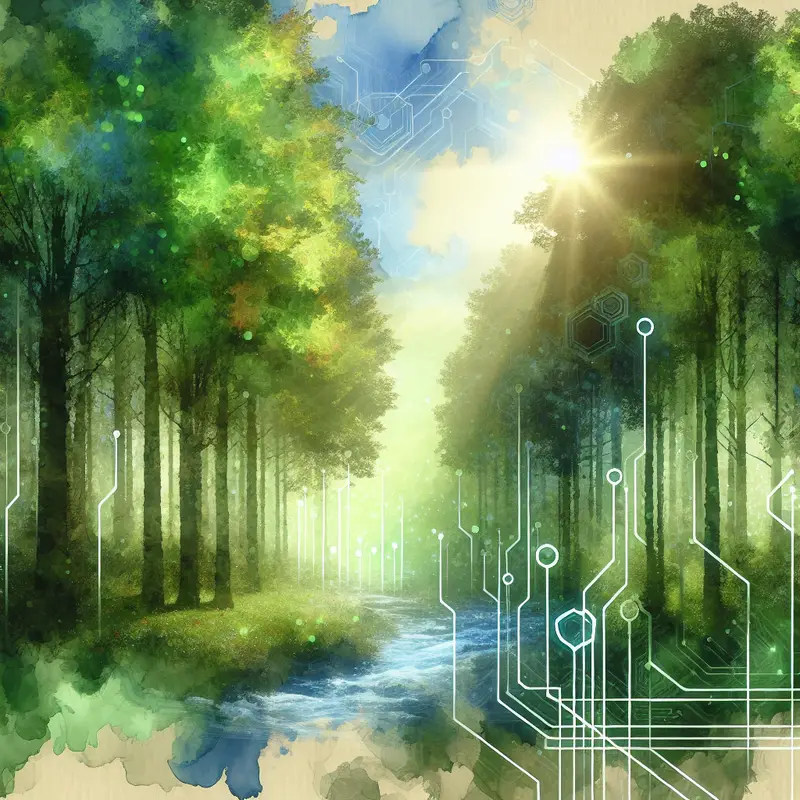
The Scale of AI's Environmental Impact
AI's remarkable capabilities come at a substantial environmental cost. Training large models like GPT series consume massive amounts of energy—around 1,287 MWh, equivalent to powering an average U.S. home for over 120 years. GPT-4, the next generation model, demands roughly 40 times more energy.
Globally, data centers hosting AI services already account for approximately 1–2% of total electricity usage, projected to rise to 3% within a few years. By 2027, AI alone could consume between 85–134 TWh annually—comparable to countries like Sweden.
Comparing AI to Other Technological Footprints
AI's per-operation energy consumption significantly exceeds traditional computing tasks. An AI query can use 10–100 times the energy of a standard Google search. Compared to blockchain technology, AI is rapidly approaching similar energy scales. For example, Bitcoin mining consumes around 100–120 TWh annually, with AI's usage quickly catching up.
While AI currently contributes 2–4% of global greenhouse gas emissions, transportation remains substantially higher at approximately 27%. However, the swift growth in AI usage is raising concerns about its future impact.
Positive Impacts: AI as an Efficiency Catalyst
Despite its significant energy footprint, AI has tremendous potential to enhance resource efficiency in various sectors. Through predictive analytics and smart optimization, AI can reduce waste, optimize logistics, enhance grid efficiency, and improve resource allocation across industries. In theory, widespread adoption of AI could yield substantial net environmental benefits by significantly cutting overall consumption and emissions.
Unintended Consequences: Rising Demand and Expectations
Ironically, AI's efficiency gains might lead to increased demand and resource use overall. As expectations for rapid, AI-driven services grow, society may inadvertently expand rather than reduce resource usage. The widespread proliferation of AI-enabled devices could exponentially amplify energy and resource consumption, negating efficiency gains achieved through smart optimization.
Beyond Electricity: Resource Demands of AI
AI's environmental impact goes beyond energy consumption:
- Water Use: AI data centers require immense water for cooling systems, potentially withdrawing between 4,200–6,600 billion liters annually by 2027.
- Material Extraction: Production of specialized AI chips requires scarce minerals like tungsten, palladium, and cobalt, leading to habitat destruction and ethical concerns surrounding mining practices.
- Manufacturing: The fabrication of semiconductor chips is resource-intensive, using significant amounts of water and hazardous chemicals.
The E-Waste Challenge
The rapid pace of AI hardware innovation results in substantial electronic waste (e-waste). AI hardware quickly becomes obsolete, with millions of tons of discarded components projected by 2030. Recycling rates remain low, and improperly handled e-waste poses severe environmental hazards through toxic contamination of soil and water.
Engineering Solutions and Mitigations
Addressing AI’s environmental impact requires strategic solutions:
- Algorithmic Efficiency: Techniques such as model pruning and knowledge distillation drastically reduce energy requirements.
- Energy-Efficient Hardware: Optimized AI chips like TPUs and GPUs significantly lower energy consumption per operation.
- Lifecycle Management: Modular hardware design and robust recycling initiatives can greatly reduce e-waste.
- Renewable Energy Infrastructure: Utilizing renewable energy in data centers drastically cuts carbon footprints. Major providers are already moving toward 100% carbon-free energy by 2030.
Stepping Off the Local Maximum: Towards Greater Efficiency
The current inefficiencies of AI may represent a temporary phase—a necessary step off the local maximum—to reach higher peaks of efficiency. Historically, technology has exhibited similar patterns: initial inefficiency gradually refined over time through innovation. Emerging solutions, such as photonic processors and neuromorphic computing, offer promising paths toward substantially reducing AI's environmental footprint.
Conclusion: A Balanced Approach
AI’s growing environmental footprint is undeniable, yet innovation promises more efficient, sustainable futures. Engineers, developers, and stakeholders must prioritize efficiency and sustainable practices to ensure that AI’s rapid advancement benefits society without compromising the environment. Through thoughtful design and sustainable infrastructure, AI can continue to revolutionize industries responsibly and sustainably.