Bigger Isn’t Always Better: The Paradox of Smarter AI
Published on March 05, 2025
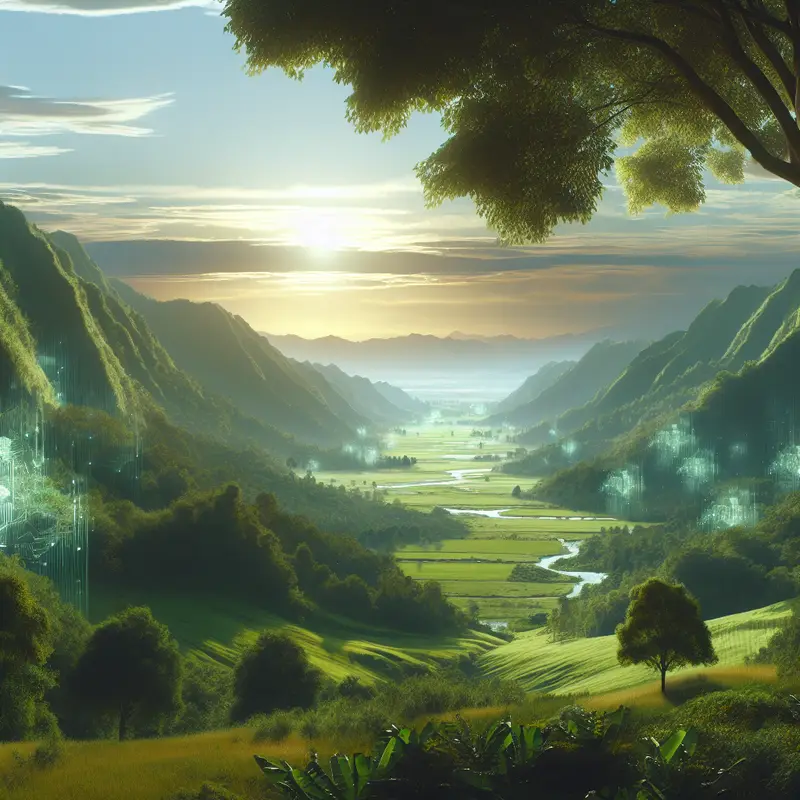
Understanding Bounded Rationality in AI
What is Bounded Rationality?
Introduced by economist Herbert Simon, bounded rationality argues that optimal decisions are made within practical constraints, such as limited information, finite processing power, and restricted time. Once decision-making processes surpass these boundaries, complexity becomes detrimental rather than beneficial.
AI models face similar constraints. Optimal performance often occurs within a manageable scope of complexity, beyond which additional analysis introduces diminishing returns or even negative outcomes.
The Limits of Increased Complexity in AI
Benchmark Scores vs. Real-world Effectiveness
While GPT-4.5 demonstrates impressive theoretical capabilities—earning incremental improvements on academic benchmarks—the translation of these capabilities into practical scenarios is often less straightforward. Real-world tasks frequently prioritize quick, confident, and actionable responses over exhaustive, nuanced analysis. Complex models like GPT-4.5, equipped with vast inference capabilities, may unnecessarily complicate otherwise straightforward tasks, slowing response times without significant gains in accuracy or utility.
The Cost of Latency
Increased complexity directly leads to higher computational costs and latency. The practical implications of slower model responses include frustrated users, disrupted workflows, and increased operational expenses—factors that can overshadow marginal gains in theoretical model accuracy.
The "Smart" Paradox: Why Simpler AI Can Perform Better
Analysis Paralysis in AI
Larger, smarter models face their own version of "analysis paralysis," a phenomenon familiar to human decision-making: when overwhelmed by possibilities, decision-makers stall, analyzing endlessly without decisive action. Simpler, smaller AI models, such as GPT-3.5-turbo, often perform better in routine tasks precisely because their constraints force quick, straightforward solutions. Rather than hesitating among nuanced options, simpler models deliver practical, usable results more efficiently.
Confidently Wrong vs. Perpetually Hesitant
Simpler models are often “confidently right,” quickly offering clear and usable responses. Conversely, advanced models like GPT-4.5—though rarely "wrong" in a simplistic sense—may hesitate, second-guess, and complicate relatively straightforward problems, appearing indecisive or inefficient. In real-world deployments, quick, actionable outputs often surpass overly cautious precision.
Matching Model Complexity to Task Needs
Right-Sizing Your AI
Effective AI integration requires carefully aligning model complexity with task demands. While simpler models are preferable for tasks like answering FAQs or managing straightforward customer interactions—due to cost efficiency and faster response times—complex models have their place, particularly in areas demanding extensive reasoning, precision, and high-stakes decision-making (e.g., legal analysis or strategic forecasting).
Practical Example
A customer-service chatbot designed to respond instantly to common inquiries may perform far better using a simpler, faster model like GPT-3.5-turbo. On the other hand, advanced legal or medical applications that demand careful, nuanced reasoning justify the additional latency and cost associated with more sophisticated models like GPT-4.5.
Stepping Beyond Bounded Rationality: When Complexity Is Worth It
Understanding the Threshold
Occasionally, the complexity barrier of bounded rationality must be intentionally breached. High-stakes decisions, ethical scenarios, or complex strategic analyses require deeper insight, broader context, and more nuanced judgment—areas where additional complexity genuinely pays dividends.
Stepping off a local maximum—intentionally embracing temporary setbacks in latency, cost, or even usability—is often necessary to progress toward higher peaks. Thus, models like GPT-4.5, despite current drawbacks, represent essential stepping stones toward Artificial General Intelligence. They allow exploration of higher-dimensional inference and understanding, even if immediate results seem counterintuitive.
Leveraging the Paradox: The Integration Specialist's Role
Knowing When to Simplify and When to Deepen Complexity
The critical role of the AI Integration Specialist is to balance complexity strategically. Specialists must recognize when simpler models suffice—and when embracing greater complexity provides genuine long-term benefits. They must clearly communicate trade-offs to stakeholders, manage expectations realistically, and ensure chosen AI solutions align precisely with business needs and contexts.
Conclusion
Bigger, smarter AI models aren’t always immediately beneficial. The paradoxical trade-offs revealed by GPT-4.5 illustrate a necessary hurdle on the path toward more advanced AI solutions. By understanding the principle of bounded rationality, integration specialists can better navigate these complexities, applying the right AI solutions effectively, confidently, and purposefully.
Ultimately, appreciating this paradox—and intentionally managing complexity—is essential to successfully deploying AI today and paving the way toward the powerful capabilities of tomorrow's AGI.