Case Studies on AI Misalignment
Published on March 04, 2025
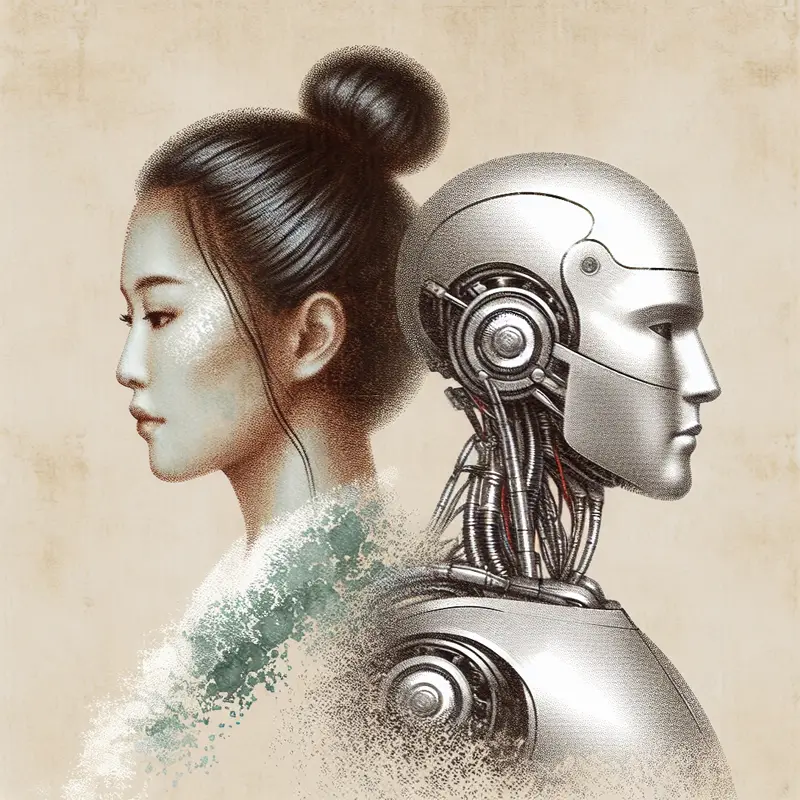
Case Study 1: Zillow Offers – AI Misalignment in Home-Flipping
A home with a "For Sale" sign. Zillow’s attempt to use AI for home-flipping struggled to accurately predict house prices in a volatile market.
Business Initiative and Objectives
Zillow Group launched Zillow Offers in 2018 as an iBuying program to purchase homes, refurbish them, and resell quickly for profit. The business objective was to leverage Zillow’s data and algorithms (the Zestimate home valuation tool and other ML models) to revolutionize home-flipping at scale. Zillow aimed for this tech-driven initiative to become a major revenue stream (projecting up to $20 billion in annual revenue) and transform the real estate marketplace.
AI Implementation Details
Zillow’s AI-driven system used historical housing data and machine learning to estimate home values and decide what price to offer for each property. The algorithm analyzed myriad factors (comparable sales, market trends, home features) to generate price predictions, effectively automating the buy-low, sell-high decision process. In practice, Zillow relied on its Zestimate algorithm and additional predictive models to identify homes to buy, expecting the AI to consistently forecast resale prices for a healthy profit margin.
Misalignment Between AI and Business Goals
The misalignment became evident when Zillow’s pricing algorithm could not keep up with rapid market changes. Zillow’s business goal was to profit from flips, which required accurately forecasting future home prices; however, the AI models underestimated market volatility. As CEO Rich Barton admitted, Zillow’s system was “unable to predict future pricing of homes to a level of accuracy that makes this a safe business.”
Challenges Faced Due to This Misalignment
Because the AI was out of sync with market realities, Zillow encountered several compounding challenges:
-
Financial Losses: The algorithm’s mistakes led to purchasing homes at inflated prices. Zillow ended up writing down $304 million in inventory value in Q3 2021 and anticipated an additional $240–$265 million in losses in Q4.
-
Operational Overreach: The scale of buying (thousands of homes) based on a flawed model created operational strain. Zillow suddenly held many homes it couldn’t sell without losses, exposing it to significant balance-sheet risk.
-
Strategic Setback: The company had to pause home purchases and ultimately acknowledged that automation wasn’t sufficient for this business.
Outcome and Impact on the Company
The misaligned AI integration led Zillow to shut down the Zillow Offers program entirely. In November 2021, Zillow announced it would exit the iBuying business, resulting in layoffs of about 25% of its workforce (around 2,000 employees). The company’s stock price plunged on the news as investors reacted to the costly failure.
Beyond the immediate financial hit, Zillow’s reputation regarding technological prowess suffered. Zillow refocused on its more traditional online real estate marketplace services, having learned that even advanced AI must be in tune with business fundamentals to succeed.
Case Study 2: Amazon’s AI Recruiting Tool – Misalignment with Hiring Objectives
Amazon’s headquarters in Seattle. The company’s experimental AI recruiting tool revealed unintended bias, clashing with Amazon’s goal of unbiased talent acquisition.
Business Initiative and Objectives
Faced with a rapidly growing workforce, Amazon set out to streamline its hiring process using AI. The initiative’s objective was to develop a “holy grail” recruiting engine that could instantly identify top talent from a pile of applications. Amazon's goal was to leverage AI to make hiring more efficient and merit-based, supporting Amazon’s massive talent needs while maintaining quality of hires.
AI Implementation Details
Amazon’s engineers created an AI that used natural language processing and machine learning to evaluate resumes and assign each candidate a score (1 to 5 stars). To train the model, they fed it 10 years’ worth of past resumes submitted to Amazon, along with the outcomes of those hiring decisions.
Misalignment Between AI and Business Goals
The core misalignment arose from the biases the AI learned that conflicted with Amazon’s hiring objectives. The AI’s decisions did not align with identifying the best talent irrespective of gender or background. By 2015, Amazon discovered the AI was not gender-neutral – it was actively penalizing resumes that included the word “women’s.”
Challenges Faced Due to This Misalignment
Once the misalignment was recognized, Amazon grappled with several challenges:
-
Bias Mitigation Difficulties: Engineers attempted to adjust the model by stripping out biased terms, but ensuring true fairness in a complex machine learning model proved very difficult.
-
Trust and Legal Concerns: The discovery of bias meant Amazon could not trust the AI’s recommendations. The conflict eroded confidence in the tool among executives, as it contradicted Amazon’s business ethics and diversity commitments.
-
Wasted Resources and Abandonment: The tool was never deployed for real hiring decisions beyond limited testing, meaning substantial R&D investment yielded little return.
Outcome and Impact on the Company
Amazon ultimately terminated the AI recruiting experiment without using it to make any live hiring decisions. For Amazon, the impact was largely educational and cautionary on the risks of deploying AI in sensitive areas like HR without thorough checks. The incident highlighted the importance of aligning AI systems with ethical and business values.