What AI Integration Is Not
Published on March 04, 2025
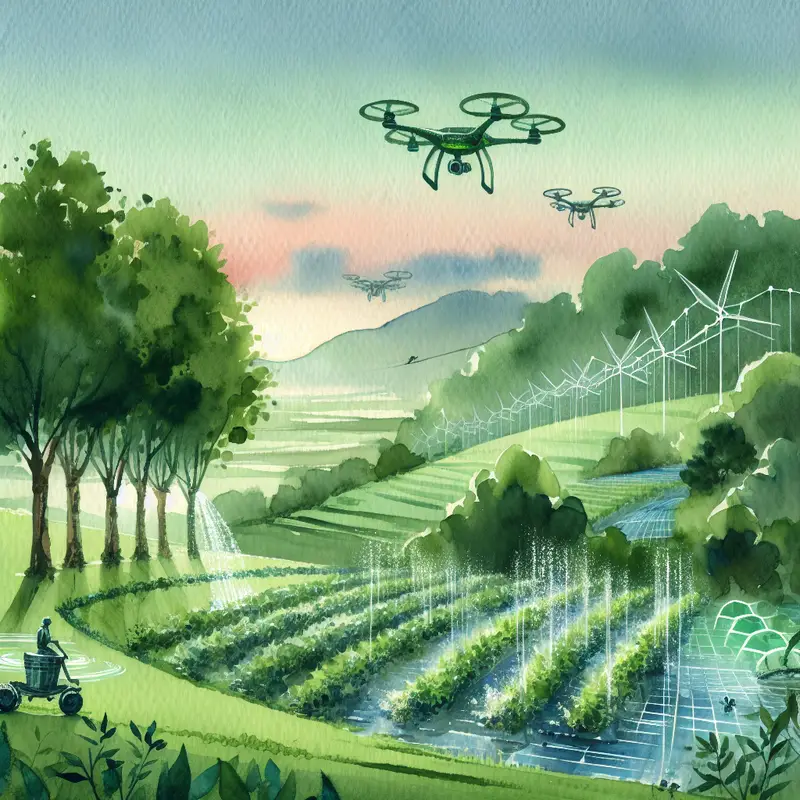
In the world of AI, there's a fierce competition to develop increasingly sophisticated models. Headlines regularly celebrate breakthroughs, new architectures, or marginal benchmark improvements. Yet, despite this spotlight on model creation, the real-world value of AI emerges not solely from building models but from integrating them effectively into practical, messy, and complex business environments.
It's critical to clarify what AI Integration is not—it’s neither pure theoretical AI research nor just model-building. Instead, AI Integration focuses on making AI solutions work effectively in real environments, bridging gaps between theoretical promise and practical success.
Beyond the Model: Integration is More Than Development
Misconception #1: AI Integration Equals Model Development
There’s a common misunderstanding that being an AI Integration Specialist means primarily developing and refining AI models. However, the reality is that AI Integration Specialists rarely, if ever, spend significant time creating models from scratch. Instead, their focus is on selecting existing proven models and effectively applying them to address specific business problems.
AI Integration Specialists must intimately understand how models work—knowing their strengths, weaknesses, and practical applicability—but their role is fundamentally about solving real-world problems, not competing for incremental gains in model accuracy.
- Model Fine-tuning: While specialists may sometimes fine-tune models for specific applications, this is distinct from foundational model-building.
- Practical Implementation: The core task is successfully embedding robust AI models into real-world business contexts.
The Limits of Benchmarks: Real-World Complexity
Misconception #2: Benchmark Success Equals Real-world Success
AI models are often tested and celebrated based on standardized benchmarks—carefully controlled environments using curated datasets. However, excelling at these benchmarks rarely guarantees successful real-world deployment. The "messy" realities of actual environments introduce complexity and unpredictability that controlled tests cannot fully replicate.
- Controlled Conditions: Benchmarks operate in idealized scenarios, with clean data and predictable tasks.
- Real-World Chaos: Actual integration requires dealing with noisy data, unpredictable user behavior, diverse software systems, hardware constraints, and complex stakeholder requirements.
For instance, a sentiment analysis model that performs perfectly on benchmark datasets might struggle when confronted with slang, sarcasm, or unique customer contexts—typical conditions it faces in live deployments.
AI Integration is Also People Integration
Misconception #3: Integration as a Purely Technical Task
Another misconception is that AI Integration is solely a technical challenge. While technical expertise is essential, the role also involves significant people management. AI Integration is as much about stakeholder alignment, expectation management, and clear communication as it is about technical execution.
- Managing Expectations: Integration specialists must clearly define and communicate what AI can and cannot realistically deliver.
- Navigating Complexity: Specialists need to be flexible, adapting quickly to unforeseen obstacles related to human or technological factors.
Indeed, integration success often hinges less on pure technical brilliance and more on skilled negotiation, effective communication, and practical problem-solving.
Solving Real-World Problems: The True Specialist Role
True AI integration is messy, iterative, and responsive to constant feedback loops. It's about delivering practical solutions that make real-world improvements—not merely theoretical gains.
AI Integration Specialists spend their days:
- Identifying genuine business problems AI can solve.
- Understanding end-user needs and workflows, ensuring integration enhances, rather than disrupts, productivity.
- Managing iterative deployments in incremental phases, adapting integration plans based on real feedback and measurable results.
- Ensuring compatibility and interoperability with existing technology, third-party software, and hardware constraints.
An integration specialist must juggle complexity from multiple directions: software conflicts, hardware limitations, user expectations, regulatory considerations, and ethical concerns.
AI Integration as People Integration
The human side of AI integration is frequently overlooked. Specialists must build trust and credibility with business stakeholders, manage resistance to change, and ensure smooth adoption. Unlike laboratory scenarios, real-world deployments must account for organizational culture, human comfort, and usability.
An integration specialist is a communicator, translator, mediator, and educator—helping businesses adopt technology effectively by guiding them through uncertainty and ambiguity.
Conclusion: Clarifying What AI Integration Really Means
AI Integration isn’t about chasing the newest model or topping benchmark leaderboards. It’s about solving tangible, real-world problems through thoughtful, practical, and often challenging integration processes. True integration specialists embrace complexity, appreciate human nuance, and thrive on bringing clarity and functionality to ambitious business goals.
As this series continues, we’ll dive deeper into practical strategies, real-world integration examples, and the diverse skills that empower specialists to make AI meaningful, impactful, and truly useful in everyday life and work.